Lung Motion Estimation
Markus D. Foote, Blake E. Zimmerman, Amit Sawant, and Sarang C. Joshi
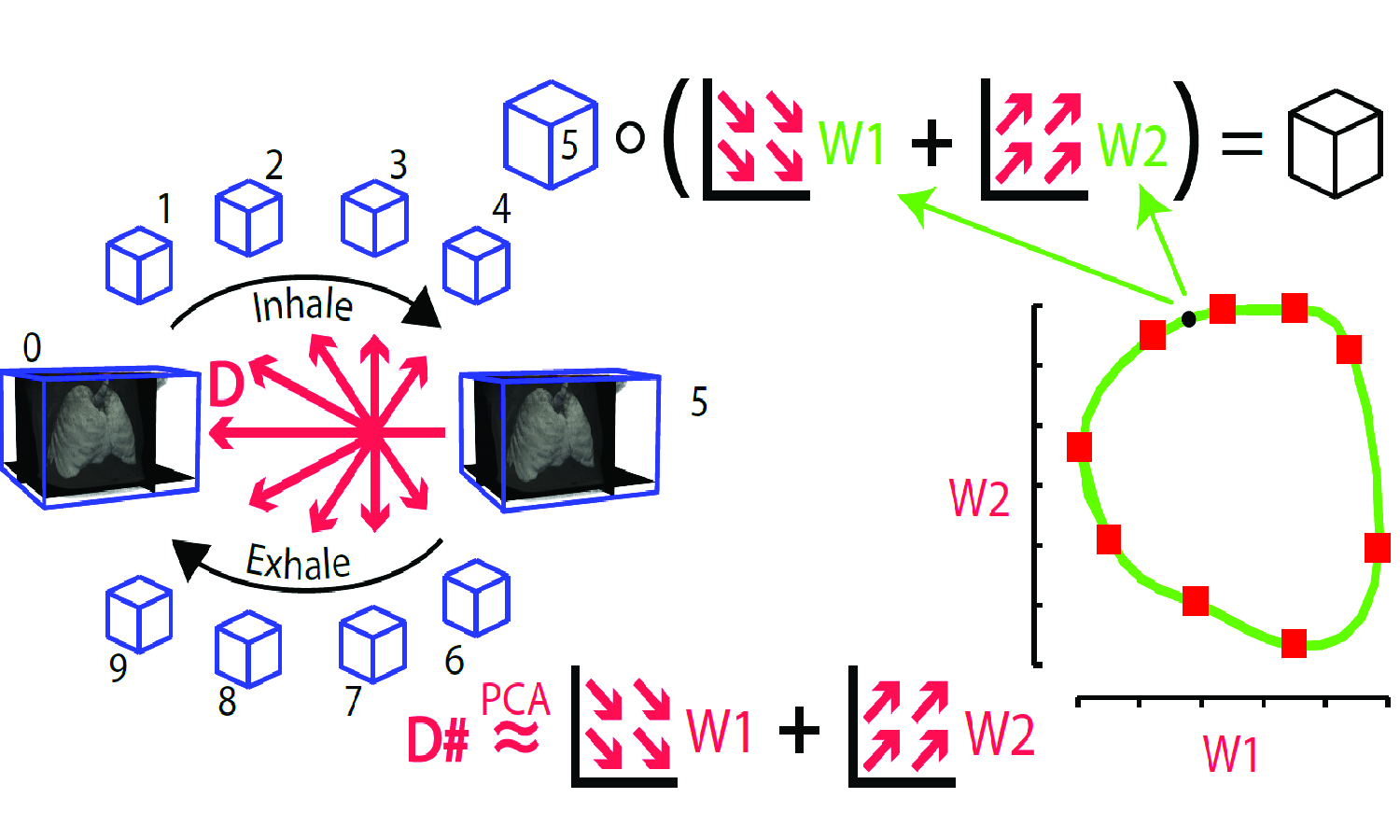
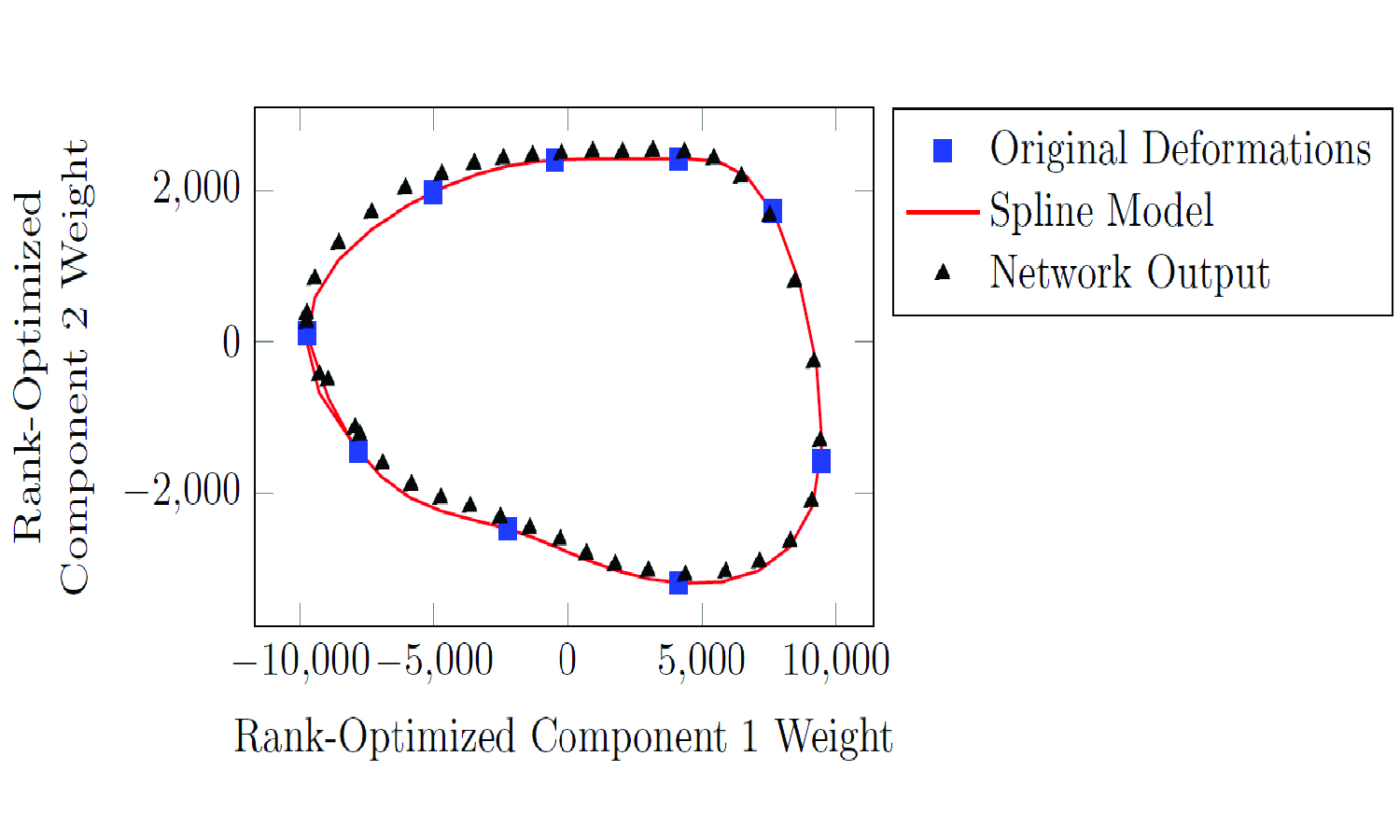
Radiation therapy for lung cancer presents a need for dynamic tracking of a target tumor volume during free breathing. Fiducial markers, such as implanted gold seeds, have been used to gate radiation delivery but the markers are invasive and gating significantly increases treatment time. Pretreatment acquisition of a respiratory correlated 4DCT allows for determination of accurate motion tracking which is useful in treatment planning (left image). In this work, we designed a patient-specific motion subspace and a deep convolutional neural network to recover anatomical positions from a single fluoroscopic projection in real-time. The deep network was used to approximate the nonlinear inverse of a diffeomorphic deformation composed with radiographic projection. This network recovers subspace coordinates (right image) to define the patient-specific deformation of the lungs from a baseline anatomic position. The geometric accuracy of the subspace deformations on real patient data is similar to accuracy attained by original image registration between individual respiratory-phase image volumes. Accurately tracking the 3D position of a lung tumor during radiation therapy can increase the dose delivered directly to the tumor and reduce radiation to healthy tissue.